DATASET
The current version of RiPPMiner-Genome has been developed using a dataset of 259 experimentally characterized RiPP BGCs (106 lanthipeptides, 55 lassopeptides, 31 thiopeptides, 26 cyanobactins, 12 glycocins, 10 LAPs, 10 head-to-tail peptides, 6 linaridins and 3 bottromycins) for which genomic sequences as well as chemical structures of RiPP biosynthetic products are known. These 259 RiPPs with BGC information contain examples where multiple RiPPs are biosynthesized by a single BGC from different RiPP precursor peptides or different types of PTMs of a single precursor peptide, thus number of unique RiPP BGCs are 204. The sequence and chemical structure information from this dataset has been used for training and validation of the machine learning (ML) classifier and benchmarking the overall performance of cross-linked chemical structure prediction starting from genomic sequences. The dataset is available in spreadsheet format as Known_RiPPs_BGC.xlsx.
BENCHMARKING RESULTS FOR RiPPMINER-GENOME
Correct identification of RiPP BGC,modifying enzymes and prediction of RiPP class could be done in 98% of cases for 9 different RiPP families. In more than 89% of cases the correct precursor peptide could be identified by the machine learning approach for these 9 RiPP families. For four major RiPP families, RiPPMIner-Genome could rank the correct cleavage and cross-link pattern among top three predictions |
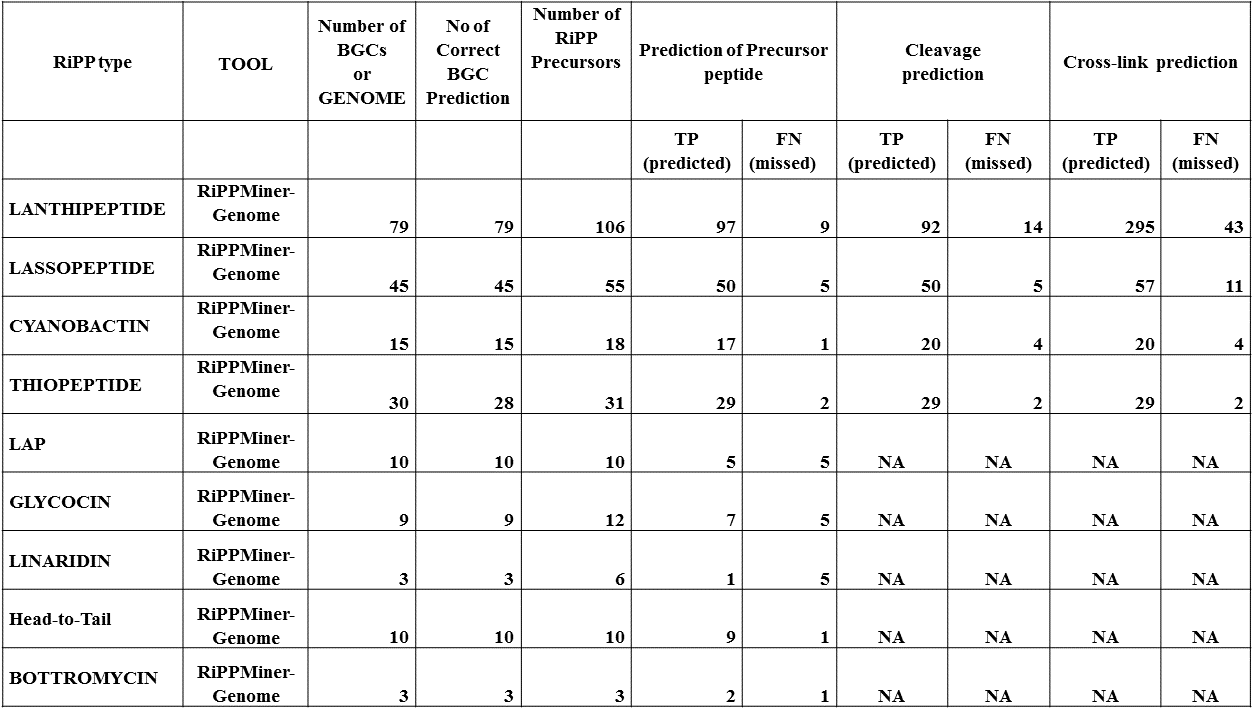
Summary of benchmarking results for predictions of RiPP precursors
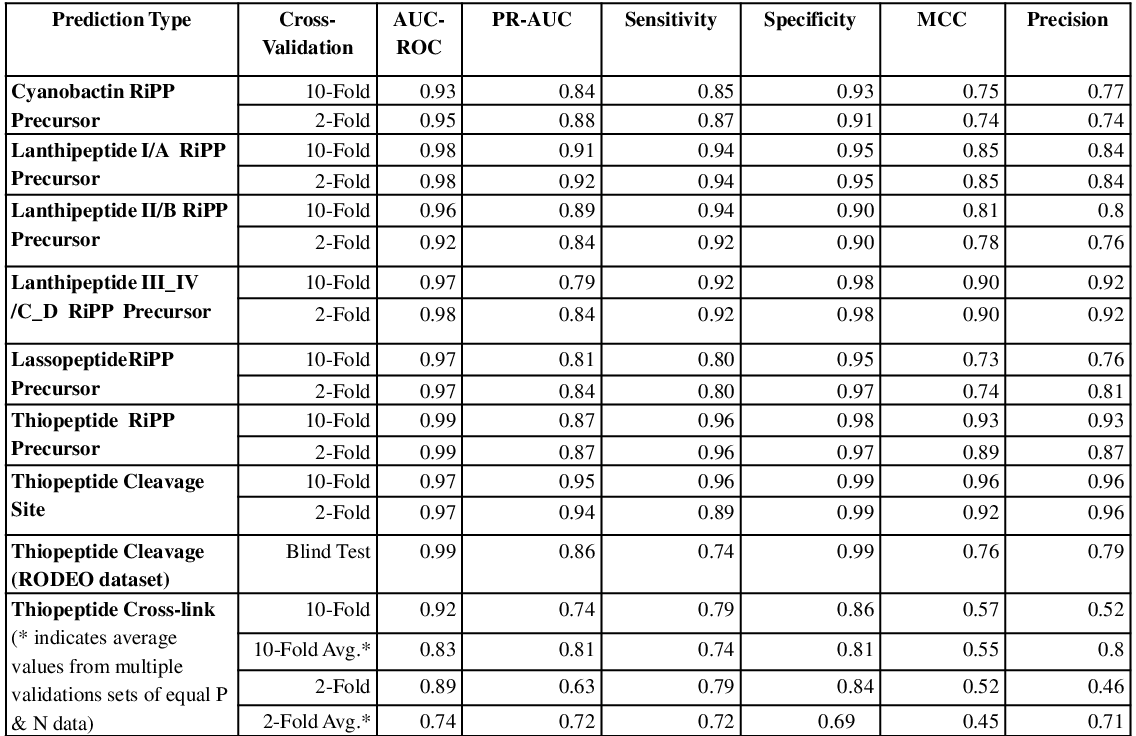
BENCHMARKING OF THE MACHINE LEARNING MODEL FOR PREDICTION OF THIOPEPTIDE CLEAVAGE
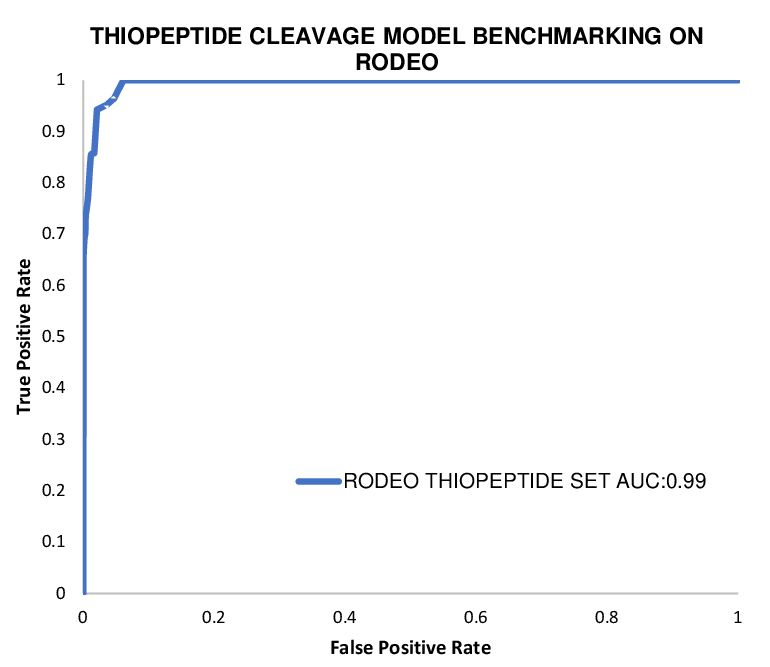
Dataset | ROC-AUC |
613 Thiopeptides(RODEO) | 0.99 |
BENCHMARKING RESULTS FOR THIOPEPTIDE CROSS-LINK PREDICTION
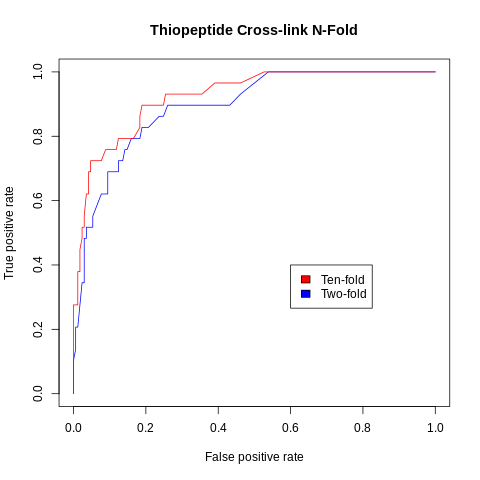
Benchmarking was carried out on the exprerimentally characterized thiopeptides BGCs.
Method: Random Forest (RF)
Total | Positive Set | Negative Set | 2-FOLD AUC | 10-FOLD AUC |
198 | 29 | 169 | 0.89 | 0.92 |
BENCHMARKING RESULTS FOR LANTHIPEPTIDE MODIFIED RESIDUES PREDICTION
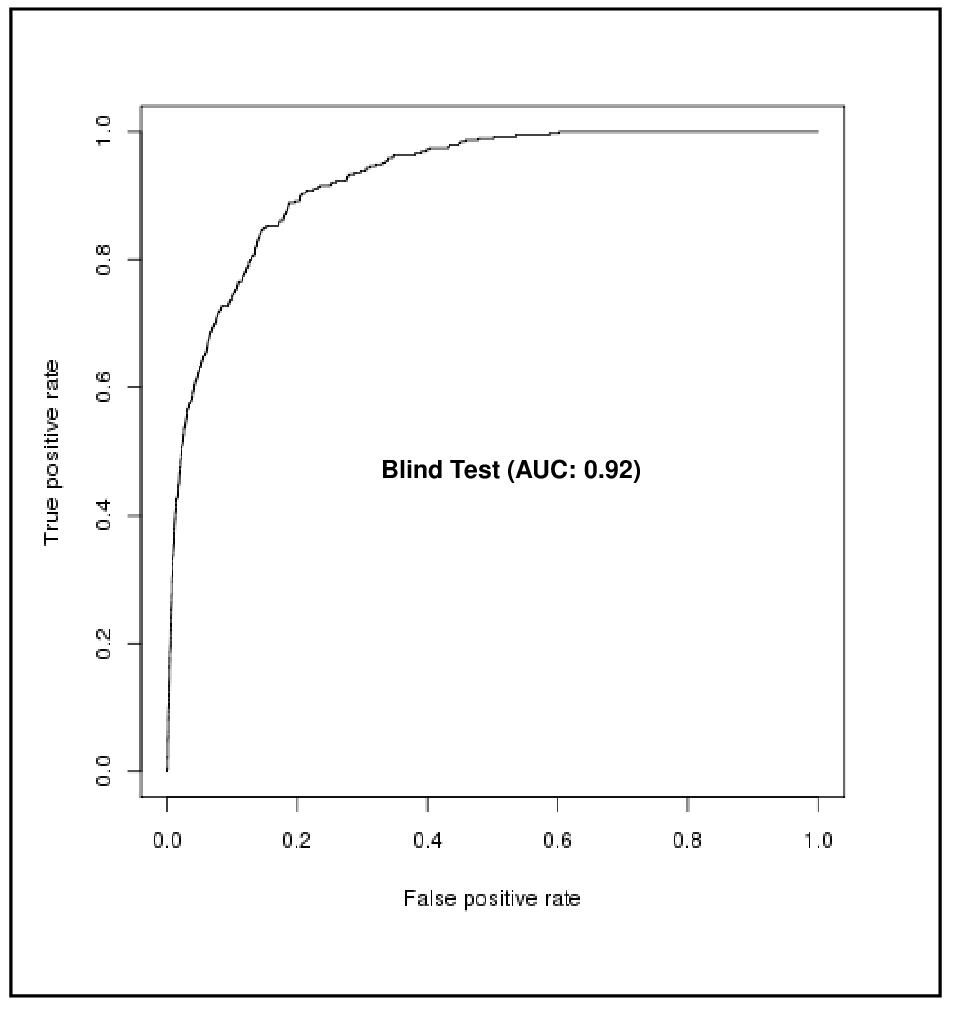
Method: Support Vector Machine (SVM)
False Positive Rate (FPR %) | True Positive Rate (TPR %) |
10 | 78 |
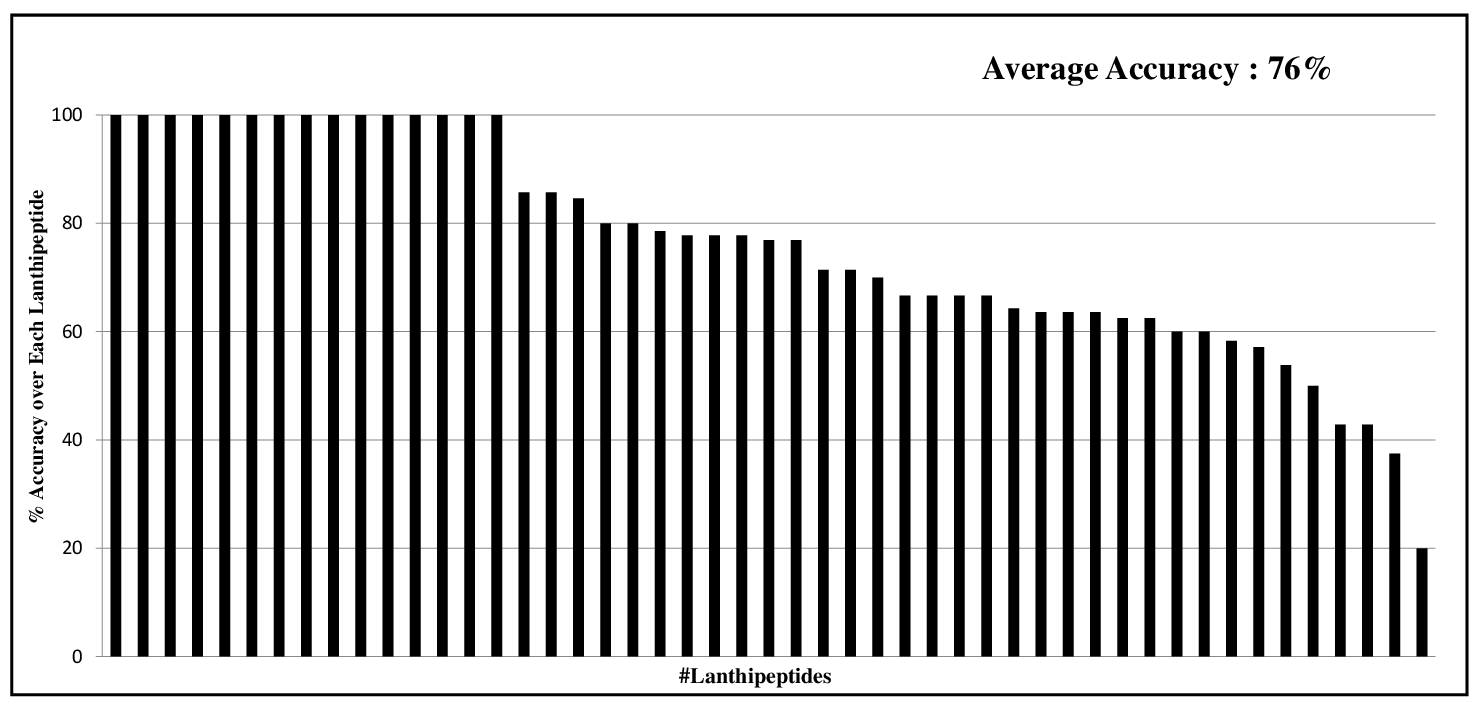
The Machine Learning (ML) Model was trained on 49 Lanthipeptides and was Tested on the remaining dataset of 49 Lanthipeptides in the Blind Test. The result shows here are the percent Accuracy for each of these 49 Lanthipeptides. The % Accuracy was calculated by counting the number of correctly predicted Modification States of Ser/Thr/Cys Residues by ML Model divided by total number of such residues present in the Core region of each Lanthipeptide. The average % Accuracy was 76%. The average % Accuracy was 76%. |
